On the Future of Continuous Monitoring for Personalized Oral Medicine (part 3)
- CORNELIU SIMA
- Aug 11, 2022
- 3 min read
Updated: Aug 13, 2022
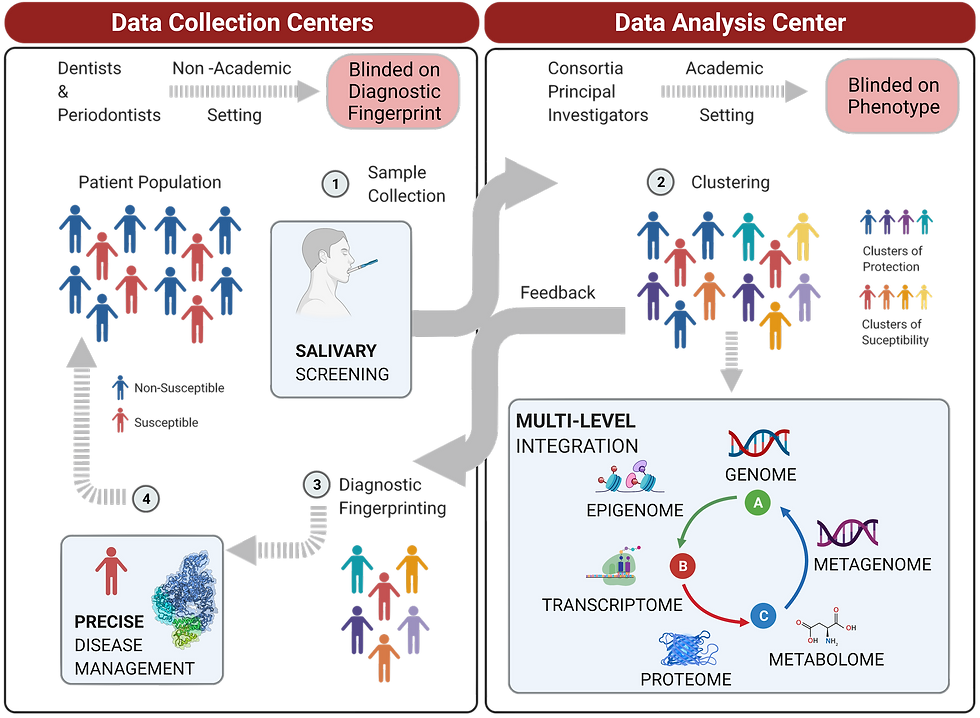
How can we harness big data/AI to make a difference in oral health? What direct impact could it have for patients?
We can harness the power of big data by developing databases and protocols for data integration using AI that will perpetually improve through ML. There are two major limitation with AI in general , including in medicine: the biases embedded in the data it is applied on and learns from, and exclusive reliance on unsupervised training, which was more common in the past. It became clearer that supervised training is necessary to generate interpretable data, which allows us to identify potential errors generated by the algorithm used. In fact supersized and unsupervised training of AI algorithms are complementary. The best examples of using exclusive unsupervised AI on data with embedded biases are in those previously used in the justice system to predict crime and those used to screen for interview candidate applying for jobs. Both have failed by biases linked to the original data it learned from.
One good example of big data integration at individual level (dense personal data) is the P100 study conducted at Univeristy of Washington to get insights into relationships among multi-omic and behavioral factors cluding whole genome sequence, clinical tests, metabolomes, proteomes and microbiomes at three time points over 9 months and daily activity tracking. The even greater value of this study is that it aims to understand what health is, which we still have trouble defining objectively. The direct impact of such an approach is that we will be able to identify individual risks for a multitude of conditions, to advance precision medicine. Patients will eventually know what they are predisposed to, how to prevent disease and what therapies are likely to work for them specifically. A good example of big data integration at population level is IBM Watson that can utilize AI to generate very useful algorithms for clinical decision making, facilitation of patient-provider interactions and improving access to care by identifying proxies to link these nodes in the chain of health care. The greatest impact on patients will likely come from the ability to personalize their disease management and better understand their unique phenotype, which should translate into better health outcomes and overall better health-related quality of life.
There are two specific limitations in big data analysis in dental medicine: the relative small scale of independent private practices collecting usable data linked to a lack of integration of this data across practices, and non-standardized protocols for data collection. The same applies to academic settings, which may not generate big data at population level independently. One good solution for this is having consortia of schools in different locations across the nation to share data into a common database. This is already implemented to some degree in the form of BigMouth consortium to which 11 schools across the US, including HSDM, contribute clinical data, at this point limited to EHR. One solution Dr. Sima is envisioning to generate big molecular oral data is to create consortia of private dental practices as satellite research centers to one or more academic institutions (Figure). Practice consortia principal investigators could then prospectively collect data including EHR, questionnaires, saliva/oral rinse, and tissue samples utilizing a standardized protocol developed in the data analysis center (academic) for subject data documentation, sample collection and transfer.
Comentários